Text generative artificial intelligences are very powerful tools. They have analyzed human works throughout history and learned how language is constructed, making them capable of creating reports, summaries, stories, articles or translations. They are also very good at identifying emotions, opinions or attitudes on social networks and other media, as well as recognizing which people, places or concepts are being talked about in each case. But despite all this, they are not good at denying hoaxes.
The reason is that human lying is more subtle. It doesn’t come apart with brute mathematical force. “These large linguistic models still have limited critical reasoning,” summarizes Iryna Gurevych, director of the Ubiquitous Knowledge Processing Laboratory (UKP Lab), one of the most influential centers in the field of natural language processing. .
Gurevych is one of the researchers who are studying how human fact-checkers behave and why AI is not capable of doing the same despite its great ability to analyze messages. “They depend too much on the existence of counter-evidence, they are incapable of reasoning about the credibility of the source of misinformation and they have difficulties detecting fallacies,” he explained in the opening talk of the European AI Conference, whose 50th edition is being held this year. year in Santiago de Compostela.
Tools like ChatGPT have had access to much of human knowledge (even that protected by copyright), but there is not always an entry in their databases that flatly denies a hoax. In the early stages of the pandemic, for example, there were no studies that concluded that bleach shots did not cure COVID-19. There was no counterevidence to that claim, but that didn’t mean the bleach did it, Gurevych said. If the misinformation is about the latest news and there is no body of knowledge about it, the AI may have too much trouble questioning it.
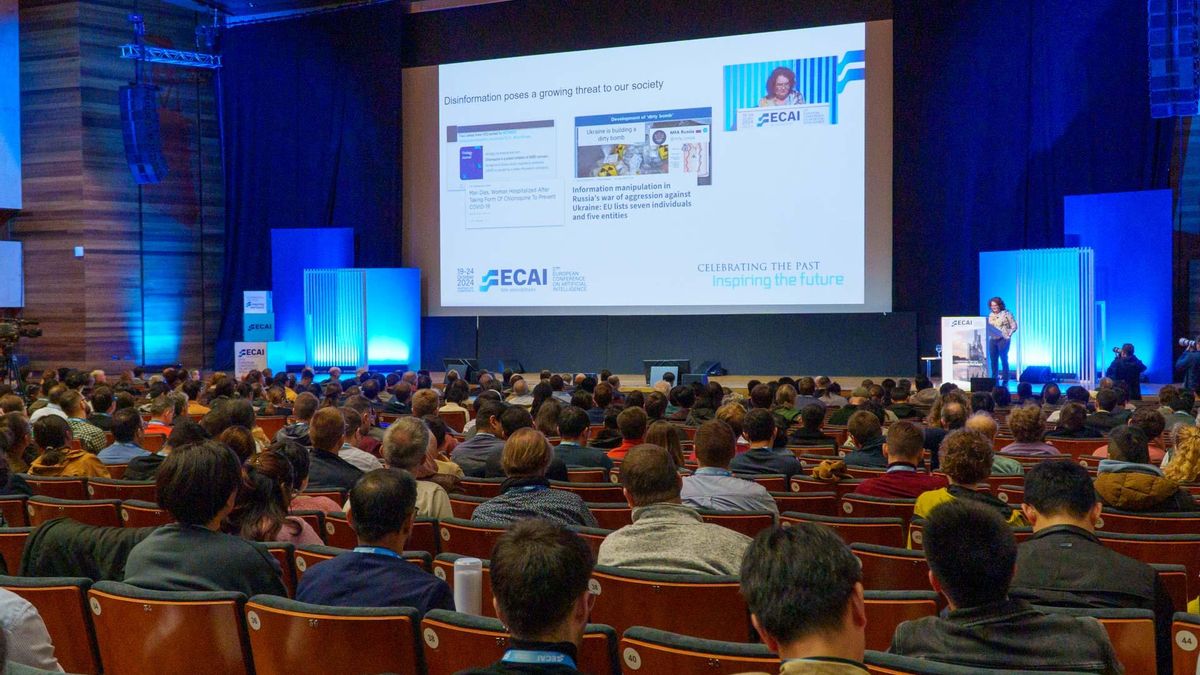
Another of its obstacles is one that humans are not free from either. Both natural language algorithms and we tend to trust data supposedly supported by scientific information or images, overlooking the fact that these may be decontextualized or misinterpreted. “People’s beliefs often do not depend on assertion and rational reasoning, but on credible content that makes the assertion seem more reliable, such as scientific publications or manipulated visual content or coming from unrelated contexts,” the professor recalled. from the Technical University of Darmstadt, in Germany.
Teaching AI to understand fallacies
To help AI tools unmask disinformers, researchers have analyzed those who know how to do it. “We look at how a denial actually works in the case of human beings. Humans have the ability to reason about a statement, even if we do not have evidence,” described the researcher at the Conference, organized by CiTIUS (Singular Research Center for Intelligent Technologies of the University of Santiago de Compostela).
“Humans will try to figure out the motivation behind the claim made by a specific entity,” he specified: “For example, we can attack the reasoning between the source and the claim. We can identify the fallacies. We can evaluate and attack the credibility of the source.” It is the same path they want to teach the AI.
To facilitate this work, Gurevych’s team has created a new data set called “Misleading” that focuses on false claims supported by scientific publications. This data set includes information on the logical fallacies commonly used by misinformers, allowing AI models to be trained to detect the patterns they follow when presenting erroneous or decontextualized evidence.
When provided with these databases, researchers have found that generative text AIs can identify fallacies with a high degree of accuracy. “We work with Llama (Meta’s AI, which will not reach Europe) and GPT. That is, the open and closed system of the great language models. And in the experiments we found that both can solve the task of detecting a fallacy given a fallacious premise objective very well,” revealed the director of the UKP Lab.
More work for images
Researchers are also trying to train AI to learn to contextualize images similar to how human fact-checkers do. Its objective is to find its original source, the date it was created, where and for what, and then contrast it with the content that you must verify.
“A fact checker uses a lot of tools in their report, such as reverse image search, where they enter the image and search for similar ones on the Internet. If there isn’t, try to describe the image with keywords and use the full standard image search to try to retrieve some content that will help them contextualize the image,” Gurevych describes. “Basically, they try to detect the time and place where the image was taken to answer the five questions: where the image came from, who created it, how, when and why.”
In order for the AI to replicate the method, they have followed the same path as with the fallacies. They have generated a data corpus with images sourced from fact-checking organisations, along with information on the five key pillars of image context.
Their results vary depending on each of these questions, but the researcher points out that “there is great room for improvement. It’s a little better for localization when we add image information. Also the image captions are quite accurate and we can fill in the details with web proofs. In future work, there are many ways we can extend the system to help human verifiers. The goal is for them to work together.”
Other bullhunting AI projects
In addition to the research presented by Iryna Gurevych in Compostela, more focused on the academic field, there are several initiatives that try to apply this technology to combat misinformation. One of them is ClaimBuster, created by the University of Texas, which uses AI to identify dubious or unsubstantiated claims.
The organization Full Fact, a UK-based fact-checking agency, uses AI to monitor content and detect disinformation automatically. “Claims are checked to see if they match something we have previously verified,” they explain.
Other tools have been created to help these professionals in more specific fields of their work. An example is Tank Classifier, which identifies what type of tank appears in an image or video; o Language Checker, which detects what language is being spoken in a given audio cut and translates it. Both have been developed at the University of Bergen with the collaboration of Norwegian verifiers.
#Artificial #intelligence #bad #debunking #hoaxes #teacher #fix